Machine Learning Intro
Machine learning is a method of data analysis that automates analytical model building. It is a branch of artificial intelligence based on the idea that systems can learn from data, identify patterns and make decisions with minimal human intervention.
Chapter1: Bird’s Eye View
Chapter2: Exploratory Analysis
The purpose of displaying examples from the dataset is not to perform rigorous analysis. Instead, it’s to get a qualitative “feel” for the dataset.
Plot Numerical Distributions
Here are a few things to look out for:
- Distributions that are unexpected
- Potential outliers that don’t make sense
- Features that should be binary (i.e. “wannabe indicator variables”)
- Boundaries that don’t make sense
- Potential measurement errors
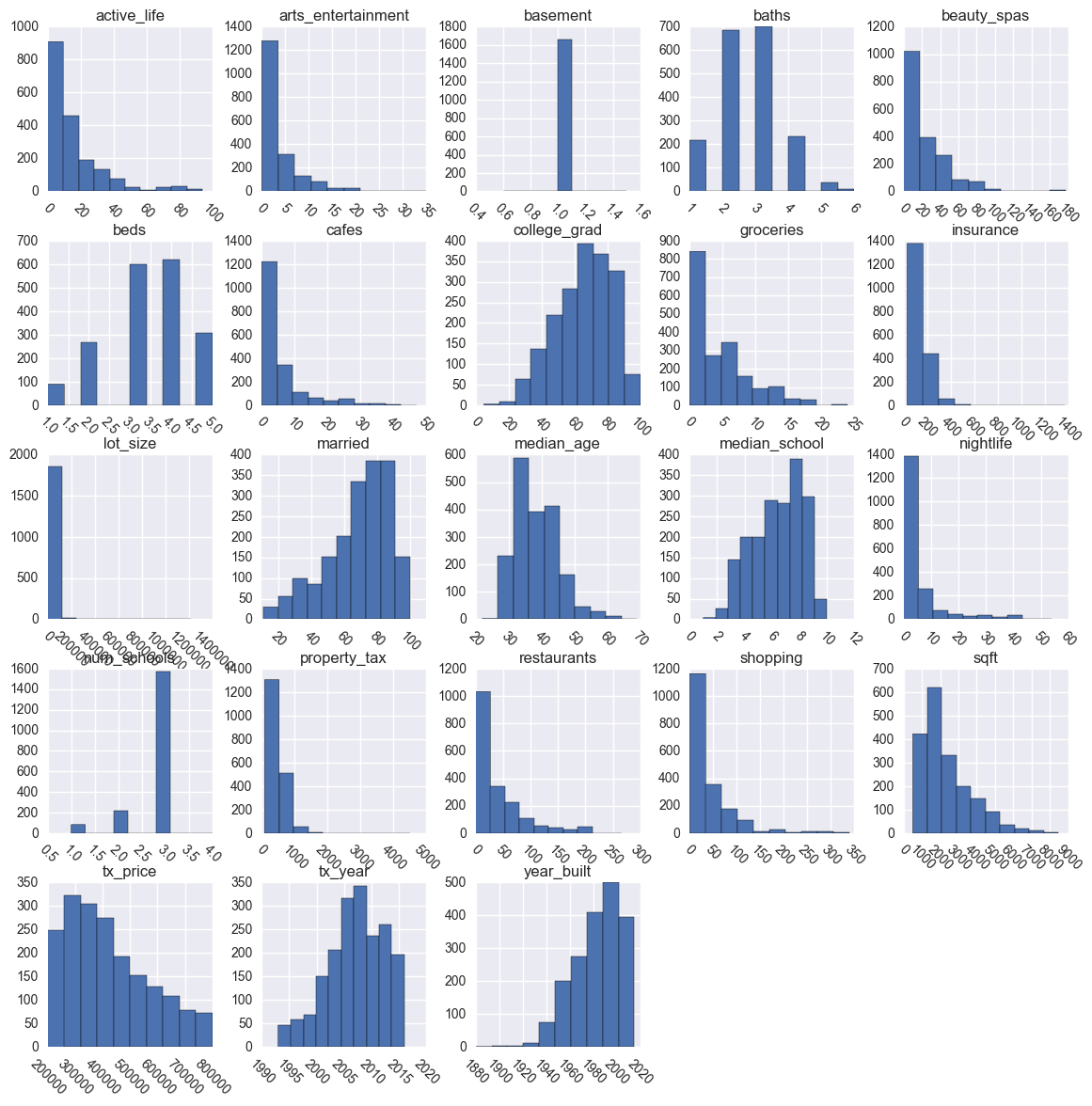
Plot Categorical Distributions
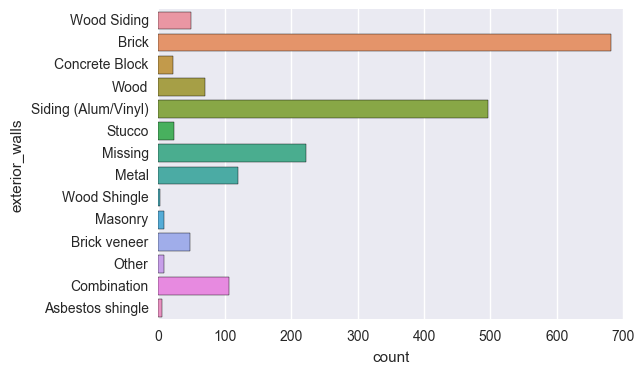
Plot Segmentations
Here are a few insights you could draw from the following chart:
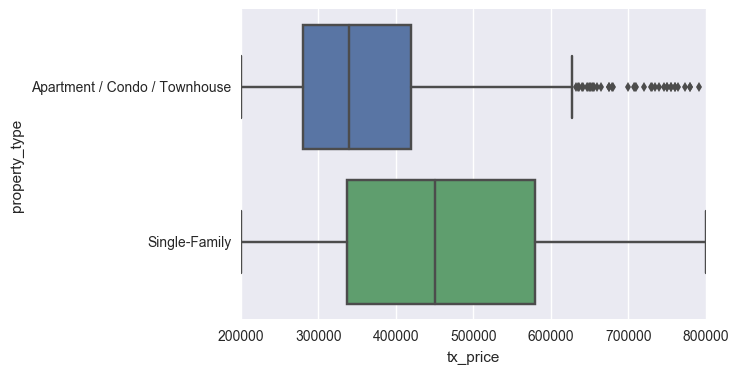
- The median transaction price (middle vertical bar in the box) for Single-Family homes was much higher than that for Apartments / Condos / Townhomes.
- The min and max transaction prices are comparable between the two classes.
- In fact, the round-number min ($200k) and max ($800k) suggest possible data truncation…
- …which is very important to remember when assessing the generalizability of your models later!
Study Correlations
- Positive correlation means that as one feature increases, the other increases.
- Negative correlation means that as one feature increases, the other decreases.
- Correlations near -1 or 1 indicate a strong relationship.
- Those closer to 0 indicate a weak relationship.
- 0 indicates no relationship.
Chapter3: Data Cleaning
Fix Structural Errors
Structural errors are those that arise during measurement, data transfer, or other types of “poor housekeeping.”
Example:
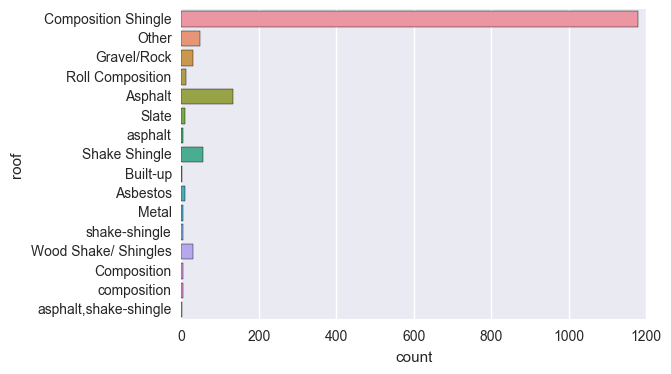
- composition’ is the same as ‘Composition’
- ‘asphalt’ should be ‘Asphalt’
- ‘shake-shingle’ should be ‘Shake Shingle’
- ‘asphalt,shake-shingle’ could probably just be ‘Shake Shingle’ as well
Handle Missing Data
Missing data is a deceptively tricky issue in applied machine learning.
the 2 most commonly recommended ways of dealing with missing data actually suck. They are:
- Dropping observations that have missing values
- Imputing the missing values based on other observations
Chapter3: Feature Engineering
Feature engineering is about creating new input features from your existing ones.
This is often one of the most valuable tasks a data scientist can do to improve model performance, for 3 big reasons:
- You can isolate and highlight key information, which helps your algorithms “focus” on what’s important.
- You can bring in your own domain expertise.
- Most importantly, once you understand the “vocabulary” of feature engineering, you can bring in other people’s domain expertise!
Infuse Domain Knowledge
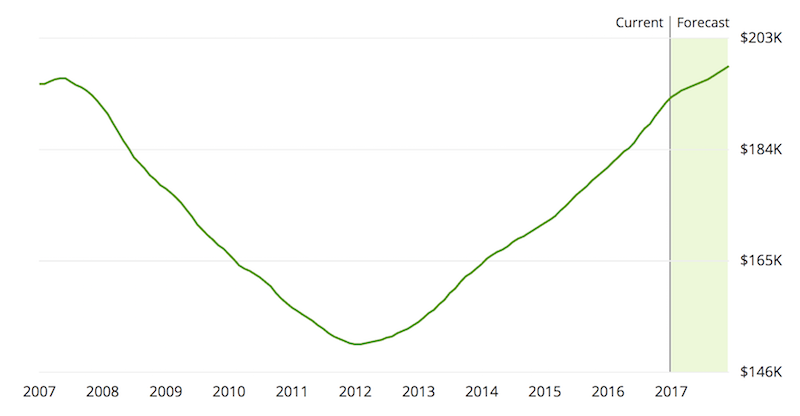
if you suspect that prices would be affected, you could create an indicator variable for transactions during that period.
Indicator variables are binary variables that can be either 0 or 1.
They “indicate” if an observation meets a certain condition, and they are very useful for isolating key properties.
Create Interaction Features
- The first of these heuristics is checking to see if you can create any interaction features that make sense. These are combinations of two or more features.
- Interaction features can be products, sums, or differences between two features.
Combine Sparse Classes
Sparse classes (in categorical features) are those that have very few total observations. They can be problematic for certain machine learning algorithms, causing models to be overfit.
Add Dummy Variables
Dummy variables are a set of binary (0 or 1) variables that each represent a single class from a categorical feature.
Chapter 5: Algorithm Selection
Why Linear Regression is Flawed
Simple linear regression suffers from two major flaws:
- It’s prone to overfit with many input features.
- It cannot easily express non-linear relationships.
Regularization in Machine Learning
Regularization is a technique used to prevent overfitting by artificially penalizing model coefficients.
- It can discourage large coefficients (by dampening them).
- It can also remove features entirely (by setting their coefficients to 0).
- The “strength” of the penalty is tunable.
Regularized Regression Algos
There are 3 common types of regularized linear regression algorithms:
-
Lasso Regression
LASSO stands for Least Absolute Shrinkage and Selection Operator.
- Ridge Regression
-
Elastic-Net
Elastic-Net is a compromise between Lasso and Ridge.
Decision Tree Algos
Decision trees model data as a “tree” of hierarchical branches. They make branches until they reach “leaves” that represent predictions.
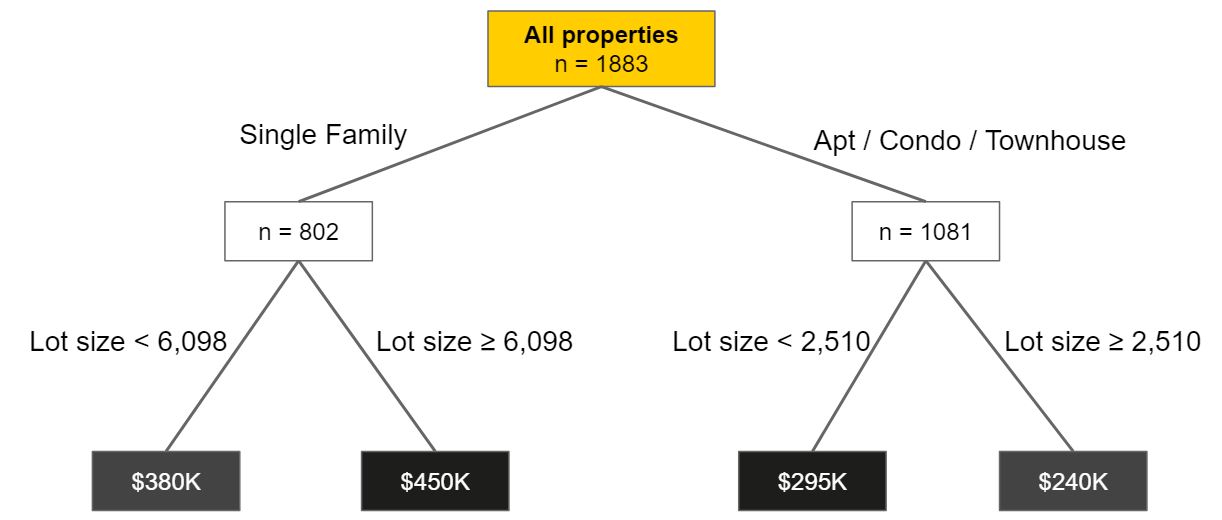
Due to their branching structure, decision trees can easily model nonlinear relationships.
Tree Ensembles
Ensembles are machine learning methods for combining predictions from multiple separate models. There are a few different methods for ensembling, but the two most common are:
-
Bagging, Bagging attempts to reduce the chance overfitting complex models.
-
Boosting, boosting attempts to improve the predictive flexibility of simple models.
Chapter 6: Model Training
How to Train ML Models
- Exploring the data.
- Cleaning the data.
- Engineering new features.
Split Dataset
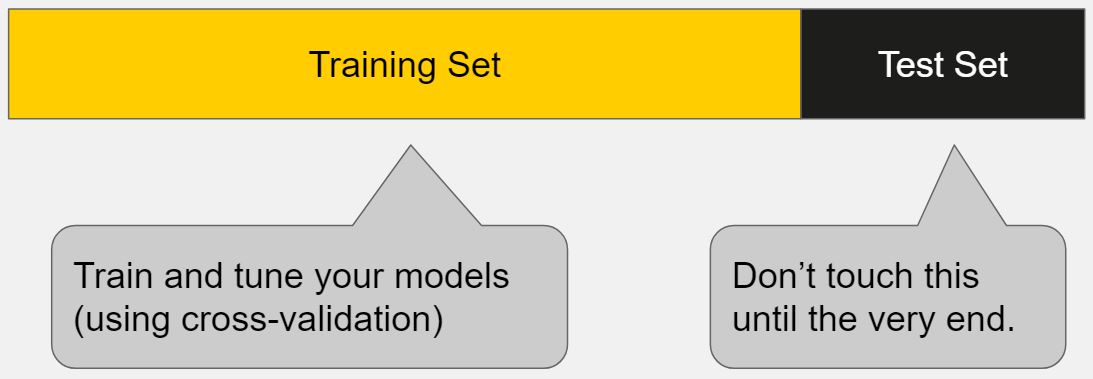
Training sets are used to fit and tune your models. Test sets are put aside as “unseen” data to evaluate your models.
Comparing test vs. training performance allows us to avoid overfitting… If the model performs very well on the training data but poorly on the test data, then it’s overfit.
What are Hyperparameters?
Model parameters are learned attributes that define individual models.such as: regression coefficients and decision tree split locations
Hyperparameters express “higher-level” structural settings for algorithms. Such as: strength of the penalty used in regularized regression and the number of trees to include in a random forest
What is Cross-Validation?
These are the steps for 10-fold cross-validation:
- Split your data into 10 equal parts, or “folds”.
- Train your model on 9 folds (e.g. the first 9 folds).
- Evaluate it on the 1 remaining “hold-out” fold.
- Perform steps (2) and (3) 10 times, each time holding out a different fold.
- Average the performance across all 10 hold-out folds.
Fit and Tune Models
At the end of this process, you will have a cross-validated score for each set of hyperparameter values… for each algorithm.
For example:
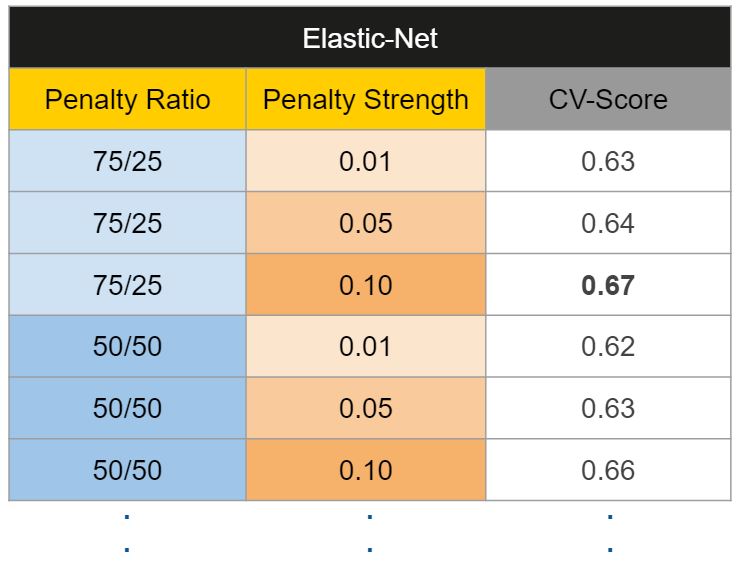
Why is machine learning important
It’s used in:
- Customer relationship management. CRM software can use machine learning models to analyze email and prompt sales team members to respond to the most important messages first.
- Business intelligence. BI and analytics vendors use machine learning in their software to identify potentially important data points, patterns of data points and anomalies.
- Human resource information systems. HRIS systems can use machine learning models to filter through applications and identify the best candidates for an open position.
- Self-driving cars. Machine learning algorithms can even make it possible for a semi-autonomous car to recognize a partially visible object and alert the driver.
- Virtual assistants. Smart assistants typically combine supervised and unsupervised machine learning models to interpret natural speech and supply context.